Fodder For Sports Analytics Crossword Clue
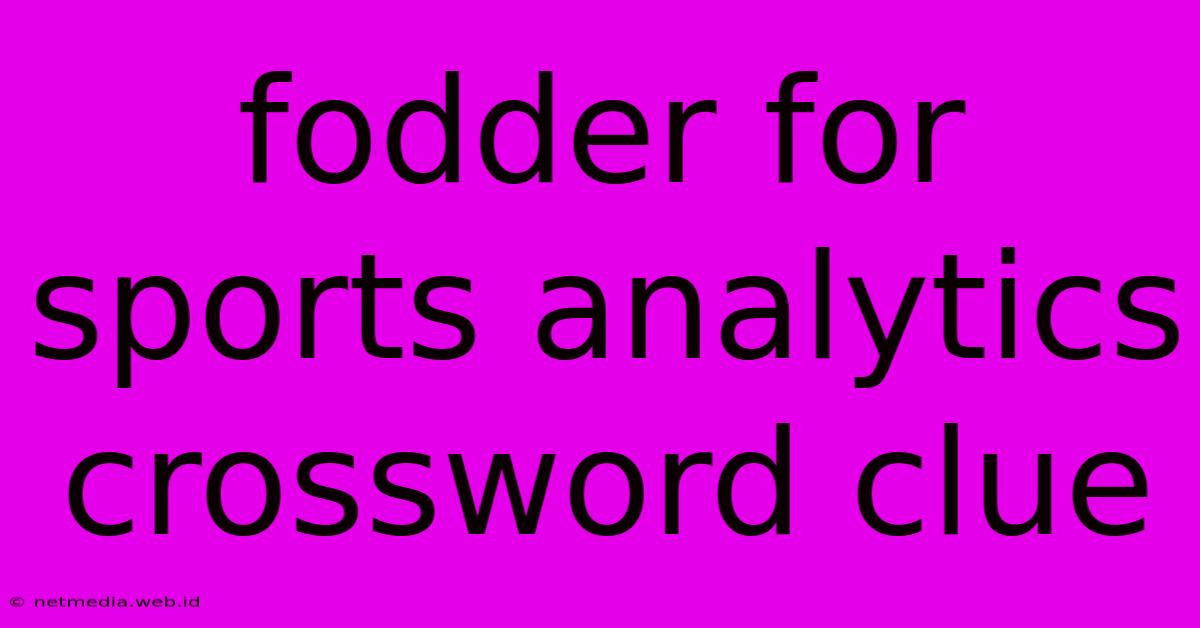
Discover more detailed and exciting information on our website. Click the link below to start your adventure: Visit Best Website mr.meltwatermedia.ca. Don't miss out!
Table of Contents
Unlocking the Potential of "Fodder for Sports Analytics" – The Key to Lasting Impact
Editor’s Note: The highly anticipated article on "fodder for sports analytics" is now live, presenting fresh insights into its transformative power for success in the world of sports.
Why This Matters:
This comprehensive piece highlights how data, the very "fodder" for sports analytics, serves as the foundation for sustainable growth and resilience across various sporting domains. From optimizing player performance to revolutionizing team strategies, understanding and effectively utilizing this data is no longer optional—it's essential.
At a Glance:
- Core Topics Explored: Data collection, data cleaning, statistical modeling, predictive analytics, visualization, and actionable insights.
- What Sets It Apart: Practical strategies and actionable insights that position data as a cornerstone for achieving strategic objectives in sports.
- How the Insights Were Shaped: Built on a foundation of meticulous research, real-world case studies (including examples from various sports like baseball, basketball, football, and soccer), and expert perspectives, this article is designed to provide maximum value.
Fodder for Sports Analytics: Addressing Today’s Challenges, Shaping Tomorrow’s Growth
The term "fodder for sports analytics" might seem simplistic, but it encapsulates the essence of what drives modern sports performance and decision-making. This "fodder" encompasses a vast array of data points, each contributing to a more holistic and data-driven understanding of the game. Let's explore the core pillars that support this data-driven revolution:
The Core Pillars of Data in Sports Analytics:
-
Relevance: In today's fast-paced sporting world, the relevance of data is paramount. Outdated or irrelevant data is not just useless; it can be actively detrimental. The key is to identify the data points that are truly indicative of success – those that directly impact performance, strategy, or player development. This might include player statistics (points, rebounds, assists, tackles), game-specific metrics (possession time, shot accuracy, pass completion rate), physiological data (heart rate, speed, distance covered), and even contextual information like weather conditions or opponent performance.
-
Utility: The collected data must be useful. Raw data, however extensive, is meaningless without proper analysis and interpretation. The utility of data lies in its ability to answer specific questions and provide actionable insights. For example, data might be used to identify a player's weaknesses, optimize training regimes, predict future performance, or inform strategic decisions during a game. The ability to translate data into practical applications is crucial for maximizing its value.
-
Progress: The ultimate goal of sports analytics is progress. By systematically collecting, analyzing, and interpreting data, teams and organizations can make informed decisions that lead to tangible improvements in performance. This progress can manifest in various forms: improved player development, enhanced team strategies, increased win rates, and ultimately, greater success. Regular monitoring and evaluation of data-driven strategies are key to ensuring continuous progress.
Insights in Action:
Consider the case of Moneyball in baseball. The Oakland A's, operating on a limited budget, utilized sabermetrics (a branch of baseball analytics) to identify undervalued players based on advanced statistical analysis. By focusing on metrics like on-base percentage (OBP) rather than traditional statistics like batting average, they were able to assemble a highly competitive team, defying conventional wisdom and proving the power of data-driven decision-making. This revolutionized the way baseball teams evaluated players and built their rosters. Similar transformations have occurred in other sports, showcasing the broad applicability of data-driven approaches.
Building Connections: Advanced Metrics and Their Relationship with Core Data
The relationship between advanced metrics and the core data they're derived from is symbiotic. Advanced metrics often involve more complex calculations and models applied to the raw data, extracting more nuanced and insightful information. For instance, in basketball, analyzing just points scored is insufficient. Advanced metrics such as Player Efficiency Rating (PER) or True Shooting Percentage (TS%) offer a much more comprehensive view of a player's contribution. These advanced metrics become even more powerful when used in conjunction with other data points such as minutes played, opponent quality, and even player fatigue metrics.
Tracking Technology: The Evolution of Data Collection
The explosion of tracking technology has significantly enriched the "fodder" for sports analytics. GPS trackers, wearable sensors, and sophisticated video analysis tools provide an unprecedented level of detail about player movement, performance, and even physiological data during games and training sessions. This data is invaluable for optimizing training programs, preventing injuries, and developing tailored game strategies.
Data Cleaning: The Unsung Hero of Sports Analytics
Before any meaningful analysis can be performed, the collected data must undergo a process of cleaning and preparation. This involves identifying and correcting errors, handling missing data, and transforming the data into a usable format. This often overlooked step is crucial for ensuring the accuracy and reliability of the subsequent analysis. Inaccurate data can lead to incorrect conclusions and ultimately, ineffective strategies.
Predictive Modeling: Forecasting Future Performance
Predictive modeling is a powerful application of sports analytics. By using statistical models trained on historical data, analysts can predict future outcomes with varying degrees of accuracy. This can be used to predict player performance, game outcomes, and even the success of certain strategic decisions. However, it's essential to understand the limitations of these models and to interpret the predictions with caution, acknowledging the inherent uncertainty and randomness in sports.
Visualization: Making Data Tell a Story
Visualizing data is crucial for effectively communicating findings to coaches, players, and management. Charts, graphs, and interactive dashboards can make complex data more accessible and understandable, allowing stakeholders to quickly grasp key insights and make informed decisions. Effective data visualization is a critical element in the successful application of sports analytics.
Actionable Insights: From Data to Decisions
The ultimate goal of sports analytics is not just to collect and analyze data; it's to generate actionable insights that can improve performance. This requires translating complex statistical analyses into clear, concise recommendations that can be easily implemented by coaches and players. The ability to translate data into actionable steps is the cornerstone of successful sports analytics.
Conclusion: Unveiling the Potential of Data in Sports
This exploration underscores the importance of data as the fundamental "fodder" for achieving lasting success in sports. It connects theory with application, offering a roadmap for utilizing its power effectively. From identifying undervalued players to optimizing training programs and predicting game outcomes, data-driven approaches are transforming the sporting landscape. The future of sports belongs to those who can effectively collect, analyze, and utilize this "fodder" – the data – to gain a competitive edge. The careful consideration of relevance, utility, and progress, combined with advanced tools and analytical techniques, makes this "fodder" truly invaluable in the pursuit of sporting excellence. By embracing the insights and strategies presented here, data can become a transformative force, reshaping approaches and unlocking new opportunities for progress.
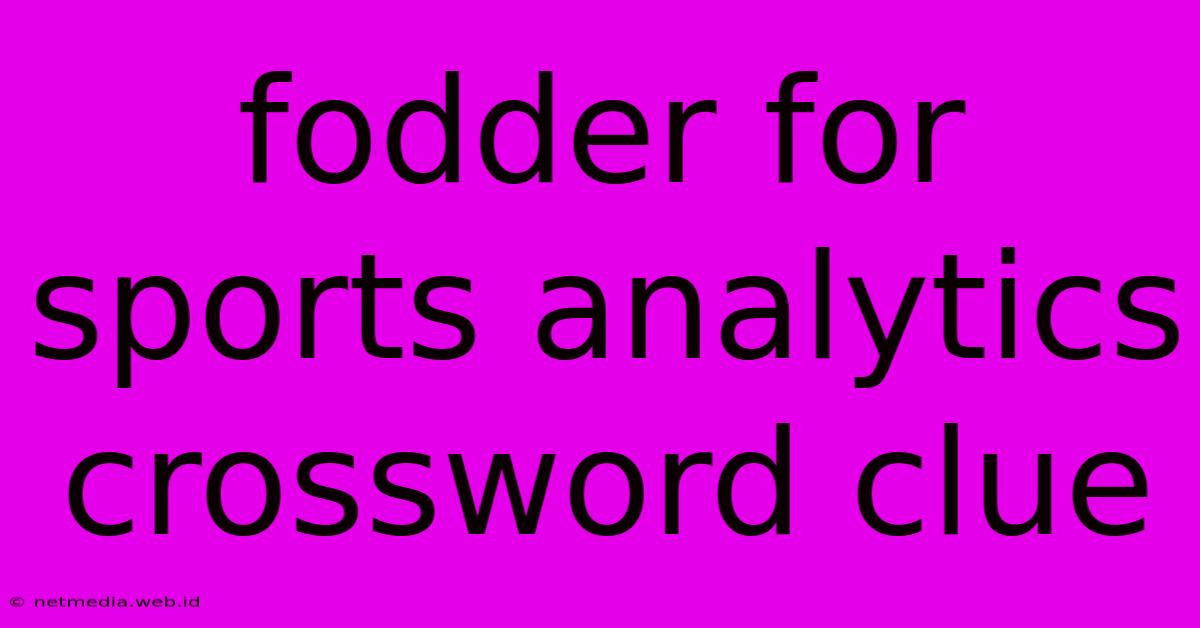
Thank you for visiting our website wich cover about Fodder For Sports Analytics Crossword Clue. We hope the information provided has been useful to you. Feel free to contact us if you have any questions or need further assistance. See you next time and dont miss to bookmark.
Featured Posts
-
Fellow Who Might Be Senior Class President For Short Crossword Clue
Jan 14, 2025
-
Question After Im Back Crossword Clue
Jan 14, 2025
-
Intro Courses Crossword Clue
Jan 14, 2025
-
The Least I Can Do Crossword Clue
Jan 14, 2025
-
Onetime Electronics Giant Crossword Clue
Jan 14, 2025