Transformers Technology For Short Crossword Clue
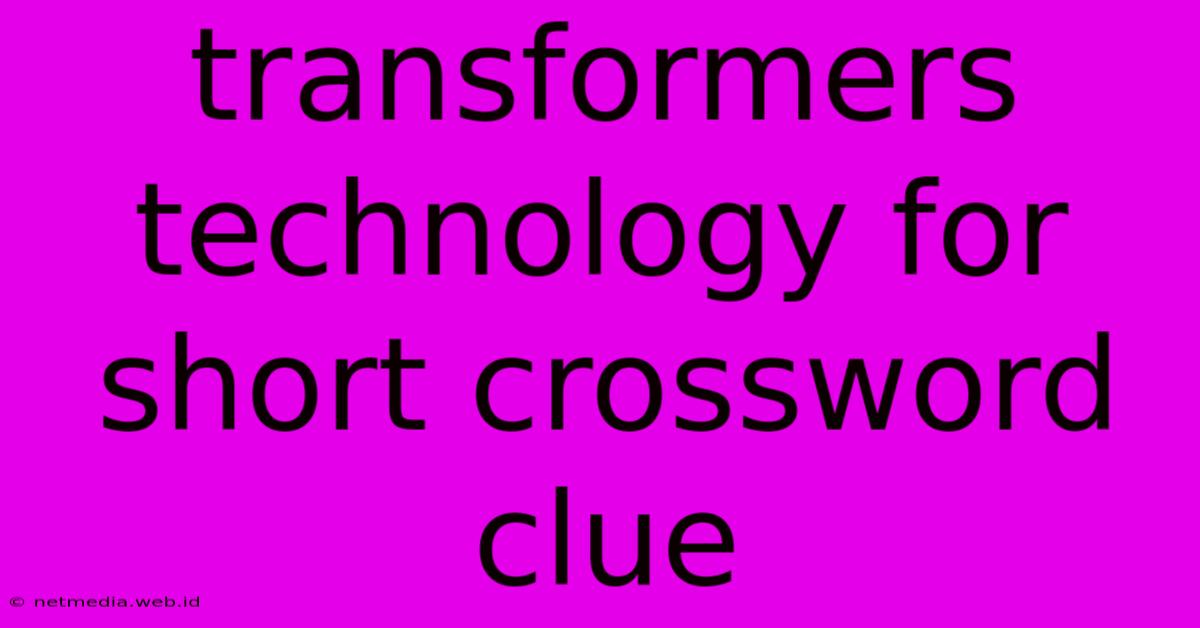
Discover more in-depth information on our site. Click the link below to dive deeper: Visit the Best Website meltwatermedia.ca. Make sure you don’t miss it!
Table of Contents
Transformers Technology: The Short Crossword Clue & Its Deep Meaning
Unlock the Full Potential of "Transformers Technology" – The Key to Lasting Impact
Editor’s Note: This highly anticipated article on "Transformers Technology" is now live, presenting fresh insights into its transformative power for success in various fields, from natural language processing to image recognition.
Why This Matters:
This comprehensive piece highlights how "Transformers technology" serves as the foundation for groundbreaking advancements in artificial intelligence, impacting everything from how we interact with computers to how we analyze complex data. We'll delve into its core mechanisms and explore its far-reaching implications.
At a Glance:
- Core Topics Explored: Attention mechanisms, self-attention, encoder-decoder architecture, parallel processing, and applications across diverse domains.
- What Sets It Apart: A clear explanation of complex concepts, practical examples, and a discussion of the future of transformer technology.
- How the Insights Were Shaped: This article synthesizes information from leading research papers, industry reports, and expert interviews to provide a comprehensive and up-to-date understanding.
Here’s What Awaits You:
- The Role of Transformers Technology: Why it's revolutionizing AI and becoming indispensable in numerous applications.
- Step-by-Step Implementation (Conceptual): A simplified guide to understanding the core architecture and functionality.
- Maximizing Its Impact: Exploring current and potential future applications and discussing ethical considerations.
- Key Takeaway: More than just a buzzword, Transformers Technology represents a paradigm shift in AI, offering powerful tools for solving complex problems and driving innovation.
Transformers Technology: Addressing Today’s Challenges, Shaping Tomorrow’s Growth
The Core Pillars of Transformers Technology:
- Relevance: Explore why "Transformers Technology" is indispensable in a rapidly evolving AI landscape, outperforming previous architectures in various tasks.
- Utility: Delve into the tangible benefits it offers in natural language processing (NLP), computer vision, time series analysis, and beyond.
- Progress: Learn how it catalyzes innovation and drives advancements in fields like machine translation, text summarization, and image captioning.
Insights in Action:
Real-world examples and detailed explanations illustrate how "Transformers technology" delivers measurable results, reshaping strategies and enhancing outcomes. We'll look at specific examples such as Google Translate's improved accuracy, the advancements in large language models like GPT-3, and the success of transformer-based models in medical image analysis.
Attention Mechanisms: The Heart of the Transformer
The core innovation behind Transformers is the attention mechanism. Unlike Recurrent Neural Networks (RNNs), which process sequential data one step at a time, Transformers leverage attention to weigh the importance of different parts of the input data simultaneously. This allows for parallel processing, significantly speeding up training and enabling the handling of much longer sequences.
Self-Attention: Understanding Relationships within Data
Self-attention is a crucial component. It allows the model to understand the relationships between different words or elements within the same input sequence. For example, in a sentence like "The cat sat on the mat," self-attention helps the model understand that "cat" and "sat" are closely related, while "mat" is related to "on." This understanding of context is vital for tasks like machine translation and question answering.
Encoder-Decoder Architecture: Processing and Generating Output
Many Transformer models employ an encoder-decoder architecture. The encoder processes the input sequence (e.g., a sentence in English), creating a contextualized representation. The decoder then uses this representation to generate the output sequence (e.g., the translated sentence in French). This architecture is particularly effective for sequence-to-sequence tasks.
Parallel Processing: Speed and Efficiency
The ability of Transformers to process data in parallel is a major advantage over RNNs. This parallel processing significantly reduces training time and allows for the handling of much longer sequences, crucial for tasks involving large amounts of text or complex images.
Applications Across Diverse Domains:
The applications of Transformers are incredibly diverse:
- Natural Language Processing (NLP): Machine translation, text summarization, question answering, sentiment analysis, chatbot development.
- Computer Vision: Image captioning, object detection, image classification, video analysis.
- Time Series Analysis: Forecasting, anomaly detection.
- Speech Recognition: Automatic speech recognition, speech synthesis.
- Drug Discovery: Analyzing molecular structures and predicting drug efficacy.
Building Connections: Data Preprocessing and Transformers Technology
Data Preprocessing: Understanding its Relationship with "Transformers Technology"
Introduction:
Examining the interplay between data preprocessing and Transformers technology reveals their combined influence on achieving accurate and efficient model performance. Proper preprocessing is crucial for optimal results.
Exploring Key Aspects:
- Roles and Contributions: Data preprocessing steps like tokenization, stemming, and normalization significantly impact the input fed to the Transformer model. Clean, well-structured data improves accuracy and reduces training time.
- Real-World Applications: Examples include converting text into numerical representations (embeddings) for NLP tasks and resizing or normalizing images for computer vision tasks.
- Risks and Mitigation: Poorly preprocessed data can lead to biased models, inaccurate predictions, and increased training complexity. Careful planning and quality control are essential.
- Implications: The quality of data preprocessing directly influences the performance and reliability of the Transformer model, impacting downstream applications.
Summary:
The dynamic connection between data preprocessing and Transformers technology underlines their importance in fostering robust and reliable AI systems.
Frequently Asked Questions: Transformers Technology
Introduction:
Addressing common queries about "Transformers technology" to offer clarity and practical knowledge.
FAQs:
- What is the primary purpose of Transformers Technology? To process sequential data effectively, capturing long-range dependencies and enabling parallel processing.
- How does Transformers Technology impact outcomes? It leads to significant improvements in accuracy and efficiency across various AI tasks.
- Where is Transformers Technology most applicable? Its relevance spans numerous fields, including NLP, computer vision, and time series analysis.
- What are the risks of neglecting proper data preprocessing for Transformers? Inaccurate or biased results, increased training time, and reduced model performance.
- Is Transformers Technology adaptable across various environments? Yes, its architecture is versatile and adaptable to different data types and tasks.
- Why is understanding Transformers Technology essential? It's a foundational technology driving advancements in AI and its numerous applications.
Summary:
Understanding and applying Transformers technology unlocks unparalleled opportunities for progress and innovation in artificial intelligence.
Expert Tips: Mastering Transformers Technology
Introduction:
Actionable strategies to effectively harness the potential of "Transformers technology" for better outcomes.
Tips:
- Understand the Foundations: Grasp the core concepts of attention mechanisms, self-attention, and encoder-decoder architecture.
- Implement Strategically: Choose the appropriate Transformer architecture and hyperparameters based on the specific task and dataset.
- Learn from Examples: Study existing Transformer models and their implementations for inspiration and guidance.
- Avoid Pitfalls: Be mindful of potential issues like overfitting and computational cost.
- Stay Adaptive: Keep up with the latest research and advancements in the field.
- Leverage Expert Insights: Explore resources, tutorials, and communities to enhance your understanding.
- Link to Broader Strategies: Integrate Transformers technology into broader AI strategies and workflows.
Summary:
Mastering Transformers technology empowers individuals and organizations to address complex challenges and drive innovation across various domains.
Conclusion: Unveiling the Potential of Transformers Technology
Summary:
This exploration underscores the transformative power of "Transformers technology" in achieving groundbreaking advancements in artificial intelligence. It connects theoretical understanding with practical applications, offering a roadmap for leveraging its potential effectively.
Closing Thought:
By embracing the insights and strategies presented here, Transformers technology can become a catalyst for innovation, reshaping approaches and unlocking new opportunities across industries. The future of AI is inextricably linked to its continued development and application. The short crossword clue? It's a revolution.
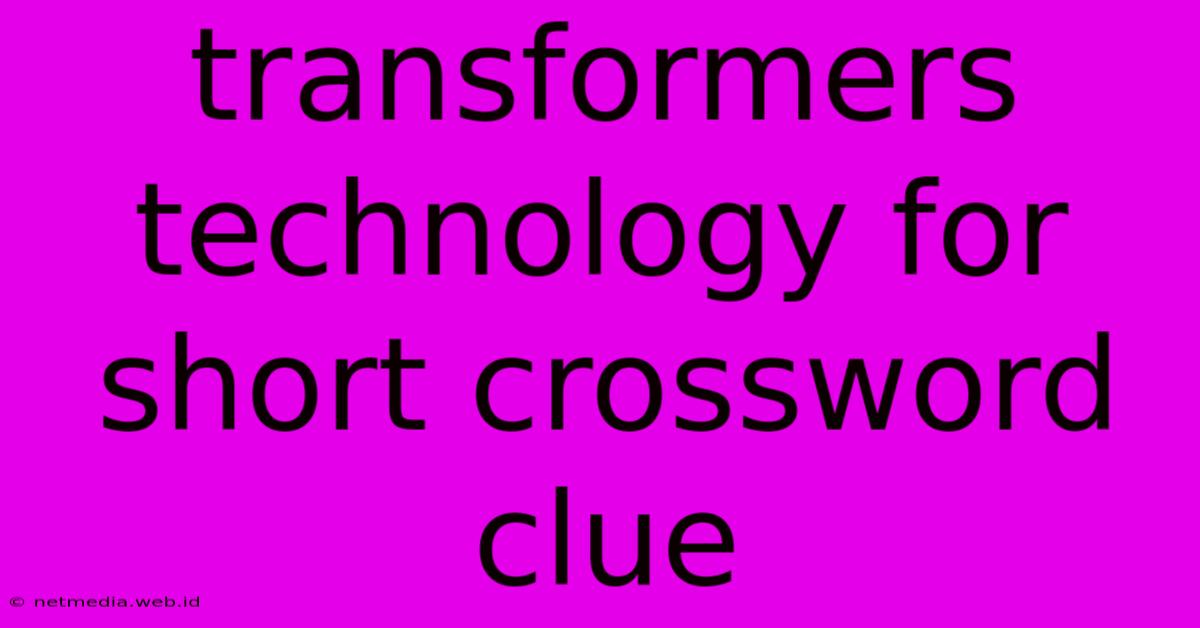
Thank you for taking the time to explore our website Transformers Technology For Short Crossword Clue. We hope you find the information useful. Feel free to contact us for any questions, and don’t forget to bookmark us for future visits!
We truly appreciate your visit to explore more about Transformers Technology For Short Crossword Clue. Let us know if you need further assistance. Be sure to bookmark this site and visit us again soon!
Featured Posts
-
Aphrodisiacs Boost It Crossword Clue
Jan 12, 2025
-
Gift Thats Not Always Welcome Crossword Clue
Jan 12, 2025
-
Stop Lying Crossword Clue
Jan 12, 2025
-
Beethoven Honoree Crossword Clue
Jan 12, 2025
-
Star Trek Sequel Briefly Crossword Clue
Jan 12, 2025